White Papers
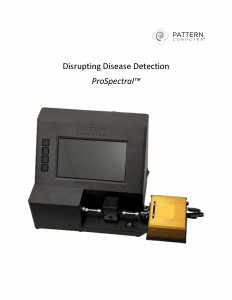
ProSpectral™ Disrupting Disease Detection
How can we better prepare for future unforeseeable viral attacks, quickly deploy broad-scale testing tools, and identify impacted communities to curtail spread as much as possible? We need a disruptive technology – one that uses different methods which provide immediate, real-time responses to mutating diseases and are free from the need for DNA replication primers or continually updated, specific antigen materials.
Pattern Computer’s ProSpectral™ instrument is one such highly innovative device. Quickly and with the accuracy of a PCR test, it can detect whether an individual is infected with a disease in only 3 seconds, as opposed to a few hours – or even days – with PCR tests. It uses no chemical or shelf-life–limited reagents; its only requirements are small polycarbonate cuvettes and two drops of saliva. ProSpectral’s science, based on advanced mathematical analysis of light patterns, will also enable devices in the field to detect a growing number of respiratory (RSV, influenza A/B) and other diseases over time, through simple software updates. The ProSpectral device is small, light, and can be battery-operated – ideal for fast and remote deployment to a local or global hotspot.
Read the paper here.
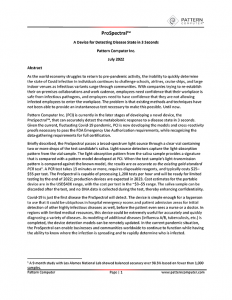
ProSpectral™
A Device for Detecting Disease State in 3 Seconds
As the world economy struggles to return to pre-pandemic activity, the inability to quickly determine the state of Covid infection in individuals continues to challenge schools, airlines, cruise ships, and large indoor venues as infectious variants surge through communities. With companies trying to re-establish their on-premises collaborations and work cadence, employees need confidence that their workplace is safe from infectious pathogens, and employers need to have confidence that they are not allowing infected employees to enter the workplace. The problem is that existing methods and techniques have not been able to provide an instantaneous test necessary to make this possible. Until now.
Read the paper here.
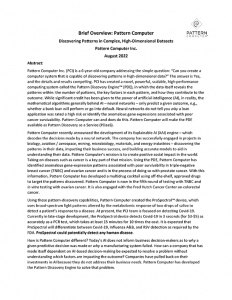
Brief Overview: Pattern Computer
Pattern Computer Inc. (PCI) is a 6-year-old company addressing the simple question: “Can you create a computer system that is capable of discovering patterns in high-dimensional data?” The answer is Yes, and the details and results compelling. PCI has created a novel, powerful, scalable, high-performance computing system called the Pattern Discovery Engine™ (PDE), in which the data itself reveals the patterns within: the number of patterns, the key factors in each pattern, and how they contribute to the outcome.
Read the paper here.
Introduction to Pattern Computer
Pattern Computer is focused on discovering patterns in complex, high dimensional datasets. Contrary to most companies using machine learning (of which most are using neural networks), Pattern Computer has developed our own proprietary algorithms to identify the critical patterns in high-dimensional datasets and are not computationally limited by the O(n2) scaling issues of neural networks (where n is the number of factors). In addition to pattern discoveries and building accurate mathematical models of the relationships between the factors, Pattern Computer also presents the relationships between these key variables in up to 8 dimensions via our Dimensional Navigator presenting the data in virtual reality. Pattern Computer is a dedicated group of approximately 20 accomplished experts in the areas of advanced mathematics, microbiology, computational algorithms, control systems, applied physics, hardware and software engineering, bioinformatics, data science, computer vision, visualization, and security.
Read the paper here.
Working with Pattern Computer
In the previously published Tech Note, “Introduction to Pattern Computer,” we provided an overview of what it is like to work with Pattern Computer Inc. (PCI). In this document, we dive deeper into that topic and address specific details regarding the process, discuss expectations for both our customers and the Pattern Computer team, and provide answers to frequently asked questions. Some parts of this discussion will be familiar from the previous document, which is good for consistency, but much more detail is provided here, with specific examples given where appropriate.
Read the paper here.
The Power of Knowing Why
Gaining insights from machine learning can be an invaluable new tool for a businessperson, technical decision maker, or researcher; it can also present new challenges. While machine-learning algorithms can reasonably predict outcomes when given new observations, as valuable as these predictions are, most algorithms can’t tell you the key factors associated with the failure of a manufacturing process, whether your loan approval algorithm is biased, or why the interaction of clinical and genetic factors results in different cancer outcomes.
How do you start to address a problem with hundreds, or even millions, of potential covariates when you don’t understand the key factors associated with an outcome? How do you gain insights into higher-order patterns?
We can do it, and we can tell you why.
Read the paper here.
What Makes Pattern Computer Different
Pattern Computer finds things that have not been found before. We find patterns in datasets that are generally too large or computationally complex for patterns to be discovered. We find what others cannot. That may be a pattern behind a specific cancer, a collection of sensor readings indicating premature part failure, or the operational patterns causing flight departure delays. Once we have discovered the key features of the pattern, we create an accurate mathematical model of the response.
Read the paper here.
Pattern Discovery: Partnering With Data Scientists
The challenge in the age of ‘big data’ is finding the meaning and insights in these large datasets. Companies and business leaders have been archiving terabytes and petabytes of information in somewhat of a cryogenic fashion waiting for the day when someone will finally unlock the patterns contained within the data and discover the insights hidden within.
Read the paper here.